
Non Covid-19 deaths by occupation – a closer look
Non Covid-19 deaths by occupation – a closer look
To download this article, please view in PDF and download from the new tab.
ONS data raises important questions about non COVID-19 deaths by occupation
Why have non COVID-19 related deaths in the hairdressing industry risen by 30%?
Following a freedom of information request on the 25th January 2021, The Office of National Statistics (ONS) released the dataset: Coronavirus (COVID-19) related deaths by occupation, England and Wales. [1]
The summary accompanying the dataset concluded that “those working in close proximity to others continue to have higher COVID-19 death rates when compared with the rest of the working age population.” [2]
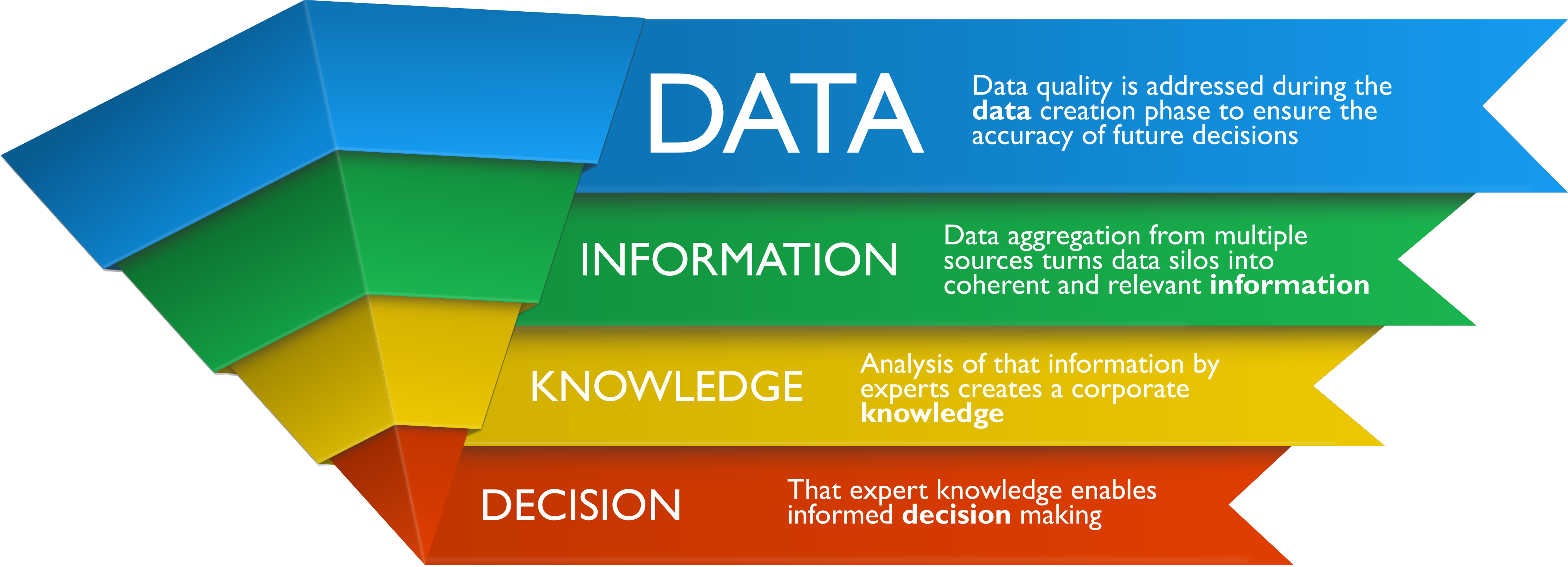
This data is clearly vital in understanding the impact of lockdown legislation on COVID-19 deaths and informs the growing conjecture about the disease’s disproportionate impact on workers with low, or irregular incomes.
Without doubt, we are fortunate in this country that the ONS provides such valuable insight to enable us to make sense of what is happening. However, the summary drew no conclusions about the increases in non COVID-19 related deaths by occupation, prompting the author to take a closer look. It highlighted a worrying increase in non COVID-19 deaths in one particular occupation – hairdressing.
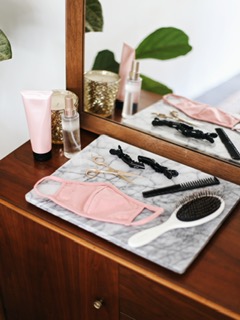
Delving deeper into the deaths by occupation data
The ONS dataset provides context to the deaths including COVID-19 against the average “expected” deaths over the same period for the past five years. [3]
The main media commentary following the release of the dataset focused on the fact that more men than women of working age had COVID-19 recorded on their death certificates. Overall, the excess deaths for women in the period covered by the dataset was 1,891. The deaths of women attributed to COVID-19 was 1,742, so no significant statistical difference. However, that total figure hides a range of outcomes across the 369 occupations listed in the dataset. When you look at the dataset in more detail some interesting numbers emerge.
In Table 1 at the end of this article (adapted from table 9 of the ONS report), I have added two extra columns: Non COVID-19 excess mortality 2020; and Percentage change Non COVID-19 excess mortality 2020.
At the “top” of the table, now sorted by percentage of non COVID-19 deaths, are hairdressers with an increase in Non COVID-19 excess mortality of 30%. But what accounts for such a marked increase and what are the leading causes of these excess deaths?
Delving deeper still – some concerning increases in several causes of death of hairdressers
Following a request for more detailed information on the mortality rate of the “top” group – hairdressers – the ONS responded very promptly on the 12th February, publishing a new dataset breaking down the leading causes of death. [4]
The total deaths, for men and women, was 398, an increase of 37% compared with the average number of deaths over the past five years covering the same reporting period. COVID-19 accounts for 20 of those deaths.
Table 2 at the end of this article (adapted from table 1 of the second ONS report) shows the top ten causes of death (out of 63) showing dramatic increases in suicide and accidental poisoning among hairdressers, as well as a startling rise in deaths from breast cancer and strokes.
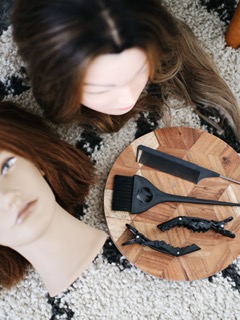
Questions we should ask next
This paper was specifically written to draw attention to a trend overlooked by most commentary on the original dataset release, namely a steep rise in non COVID-19 related deaths in certain professions, and in particular hairdressers.
As more datasets are released covering longer periods of time, new trends in the data will become apparent. It is still too early to draw definite conclusions, and whilst we must always be careful to remember that correlation does not imply causation, these datasets do raise the imperative to ask more questions such as:
- Why is it that, during this pandemic, COVID-19 was responsible for less than 7% of the 37% increase of deaths in hairdressers?
- What is driving the increase in nine of the top ten causes of deaths among hairdressers?
- Breast cancer deaths among hairdressers are up by 44%. Is this figure an outlier, if not, what is driving this increase?
- What is behind the doubling of deaths from strokes among hairdressers?
- Deaths from suicide and accidental poisoning are up nearly 50%, and together, are more than double the deaths from COVID-19. Why?
Increased deaths across this many categories in a single occupation cannot simply be dismissed as an outlier, or a one-off event. There will almost certainly be an underlying cause.
Many hairdressers are self-employed and have been unable to work for long periods since March 2020. A lot of money was spent by these businesses to make their salons safe when they reopened after the first lockdown.
There has been a lot of recent commentary in the media about how many excess deaths may have been caused as a result of the lockdown policies. Is this an early indicator of this effect? Certainly, the rises in accidental poisoning and suicides in this – generally low paid – occupation is extremely worrying.
The original dataset, published in January, lacked the context of the occupation size and the median income of each occupation. Obtaining these additional data elements may tell us more about the anecdotal evidence that it is the poor, or those with irregular incomes, who are suffering disproportionately from the lockdown. Perhaps the ONS will add these data fields to the next release.
Hopefully, the NHBF, the trade body for hairdressers, will also study this dataset in more detail and work with their membership to reduce some of the tragic, avoidable deaths in these categories.
Acknowledgement: Open data and the Office for National Statistics
We are very fortunate to have the ONS and an open data policy in the UK. I would like to thank the ONS for their prompt response to my request, and the great work they do in regularly publishing datasets that allow us to examine for ourselves what is really happening. This open data policy allows anyone to delve beyond the headlines we see every day.
Tables
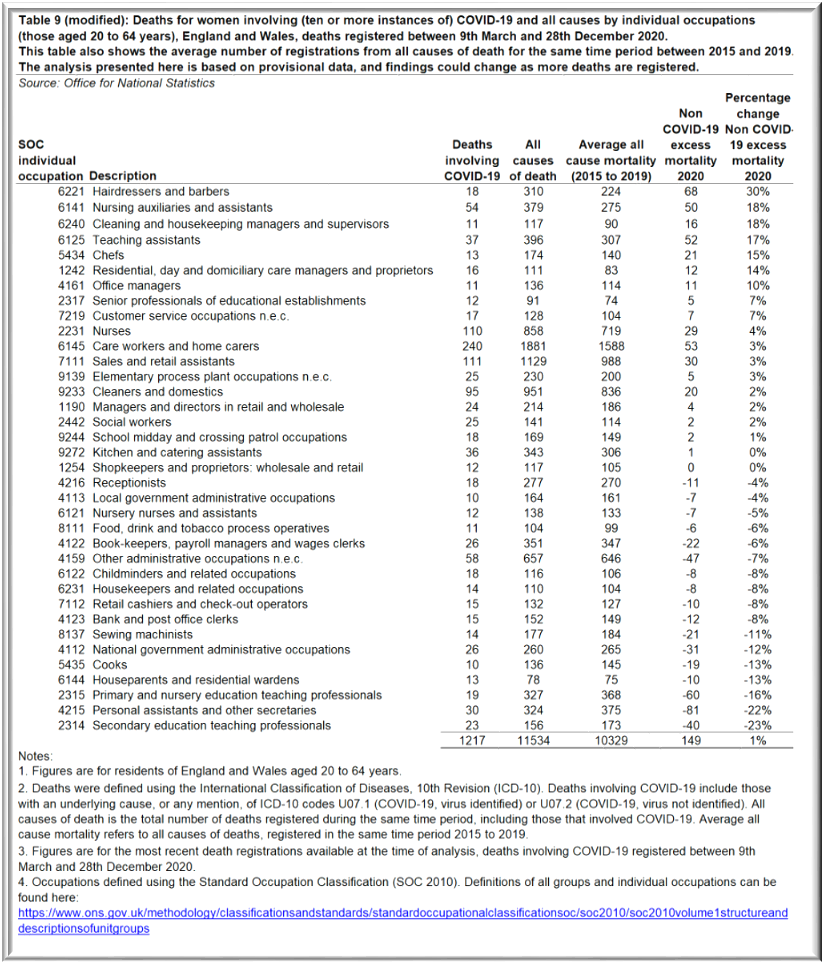
Table 1: Deaths for women by occupation involving ten or more instances of COVID-19
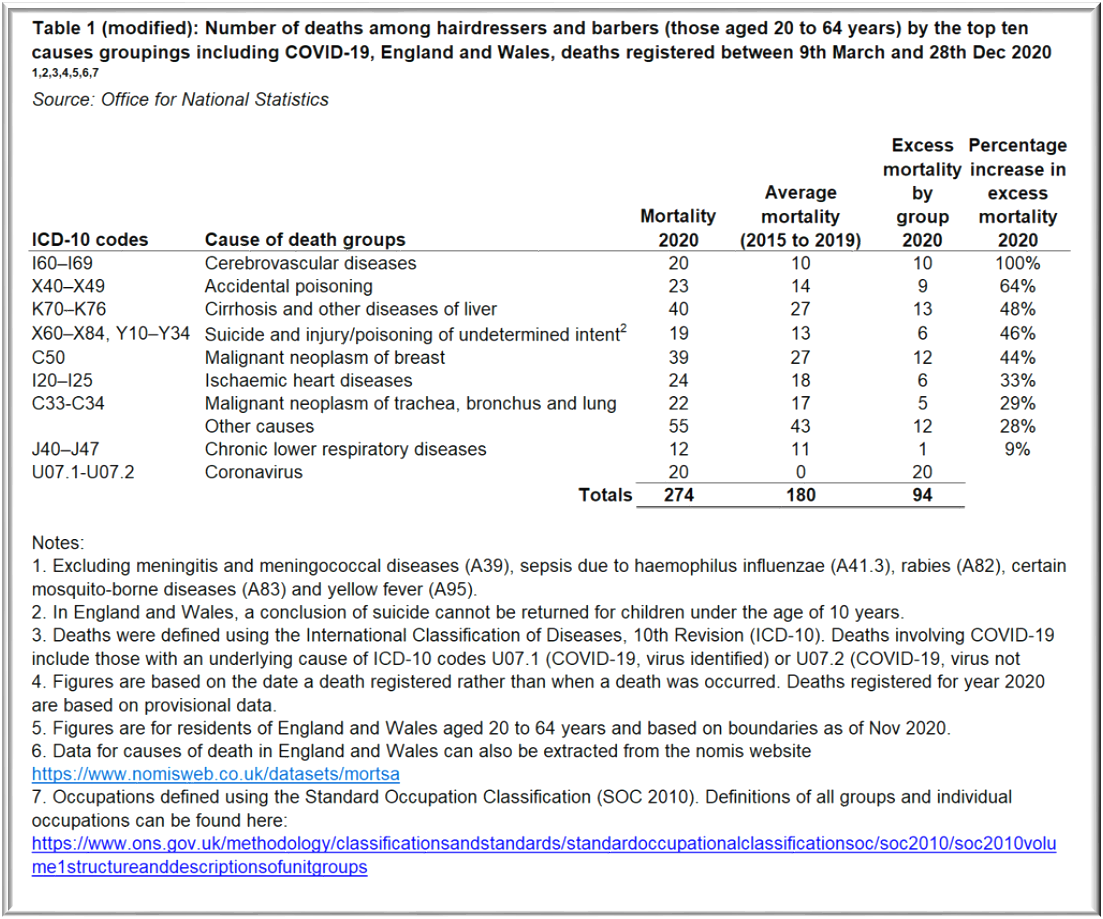
Table 2: Top 10 causes of death among hairdressers
References
[1] https://www.ons.gov.uk/peoplepopulationandcommunity/healthandsocialcare/causesofdeath/bulletins/coronavirusCOVID19relateddeathsbyoccupationenglandandwales/deathsregisteredbetween9marchand28december2020
[2] “Today’s analysis shows that jobs with regular exposure to COVID-19 and those working in close proximity to others continue to have higher COVID-19 death rates when compared with the rest of the working age population. Men continue to have higher rates of death than women, making up nearly two thirds of these deaths.”
Ben Humberstone, ONS, Head of Health Analysis and Life Events, 25th January 2021
[3] The dataset covers deaths involving COVID-19 and all causes by sex (those aged 20 to 64 years), England and Wales, for deaths registered between 9th March and 28th December 2020.
Deaths are defined using the International Classification of Diseases, 10th Revision (ICD-10). Deaths involving COVID-19 include those with an underlying cause, or any mention, of ICD-10 codes :
- U07.1 (COVID-19, virus identified) or
- U07.2 (COVID-19, virus not identified).
All causes of death is the total number of deaths registered during the same time period, including those that involved COVID-19.
Table 9 in the dataset breaks the figures down by occupation. Occupation is defined using the Standard Occupation Classification (SOC 2010). The table lists 369 occupations. Table 9 breaks the dataset down further by male and female.
The three columns of figures supplied in the dataset are titled:
- Deaths involving COVID-19;
- All causes of death;
- Average all-cause mortality (2015 to 2019)
[4] https://www.ons.gov.uk/peoplepopulationandcommunity/healthandsocialcare/causesofdeath/adhocs/12888numberofdeathsamonghairdressersandbarbersthoseaged20to64yearsbyleadingcausesofdeathsdeathsregisteredbetween9marchand28december2020englandandwales
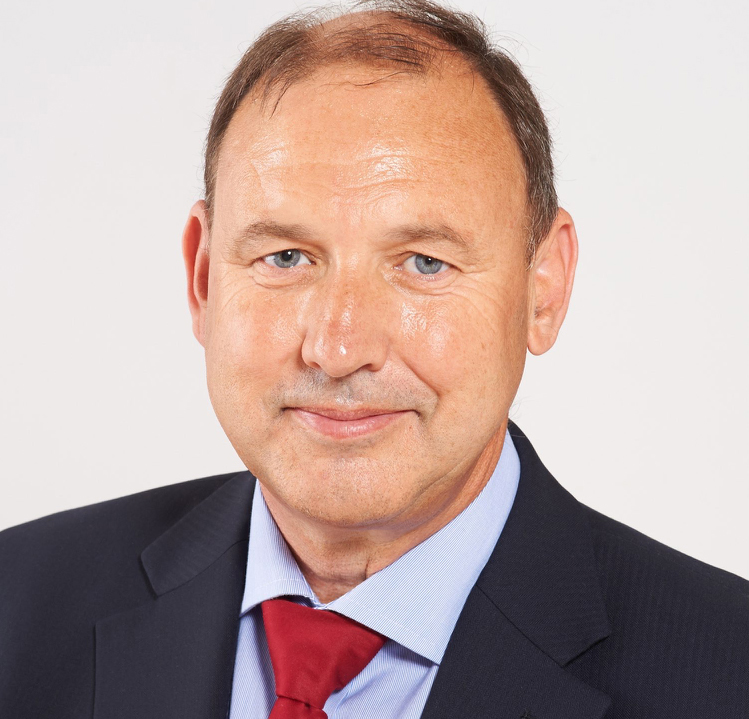
About the author
Peter Eales is chair of KOIOS Master Data, a provider of cloud-based data quality software. KOIOS also provides data quality consultancy and training services based on International Standards for data quality. Peter is an internationally recognised expert in the field of characteristic data exchange, and industrial data quality. Peter is a member of a number of International Organization for Standardization (ISO) working groups drafting International Standards in these areas.
Peter has a daughter who is a self-employed hairdresser
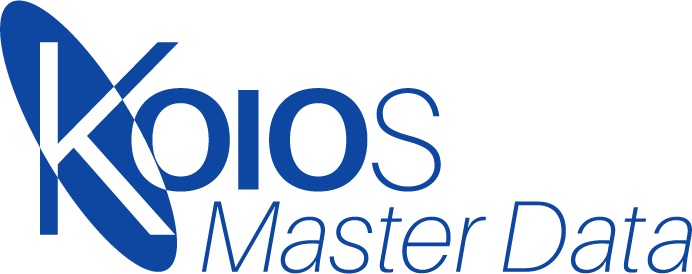
Contact us
+44 (0)23 9387 7599